Translate this page into:
The Cancer Genomic Atlas – “TO CONQUER CANCER”

*Corresponding author: Sai Sri Kavya Kadali, Department of Biochemistry, Andhra University, Visakhapatnam, Andhra Pradesh, India. kavyakadali13@gmail.com
-
Received: ,
Accepted: ,
How to cite this article: Kavya KSS, Gowlikar R, Fatima SN. The Cancer Genomic Atlas – “TO CONQUER CANCER.” Int J Mol Immuno Oncol 2021;6(2):76-81.
Abstract
The Cancer Genomic Atlas (TCGA) is a publicly accessible cancer data repository and tool that allows us to understand the molecular basis of cancer through the application of genomics and proteomics. So far, researchers have been able to diagnose 33 cancer types including 10 rare cancer types. The key features of TCGA are to make the data collection process publicly accessible for the better understanding of the molecular and genetic basis of cancer and its mechanism of action along with its prevention. Studies on different cancer types along with comprehensive pan cancer analysis have expanded the understanding and purpose of TCGA. Ever since its’ conceptualization, its’ high-throughput approach has provided a platform for the identification of genes and pathways involved in cancers and accurate classification of cancers.
Keywords
Genomics
Molecular biology
Bioinformatics
Database
Oncology
INTRODUCTION
For centuries, cancer has been affecting humans aggressively, where the first documented cases of cancer were hailed in 1500 B.C. in Egypt although the term was later proposed by Hippocrates (Father of Medicine).[1] With the advances in medical science, cancer which initially started as breast cancer has increased vastly by spreading to other organs. Cancer is generally characterized by genomic alterations such as chromosomal rearrangement, copy number aberrations, DNA sequence changes, and modification in DNA methylation which together increases the human malignancies.[1] The cell becomes cancerous when there are mutations in genes, such as the tumor suppressor p53 or TP53. In fact, more than 50% of cases are caused when there is a defect in p53. The continued uncontrolled cell division leads to accumulation of cells in the body which aggregate to form a tumor.
Fast forward to the 21st century, with strides made in molecular oncology, a recent advancement was the introduction of The Cancer Genomic Atlas (TCGA). Today, TCGA is a publicly accessible tool developed for better understanding of the molecular basis of cancer through genome sequencing. It was initiated in February 2005 by the National Cancer Advisory Board, and finally, it was released in 2006 by the joint collaboration of National Cancer Institute (NCI) and National Human Genome Research Institute (NHGRI).[2] It is a public platform which is designed to help researchers and clinicians to improve diagnostic methods, treatment standards, and cancer prevention. From the point of its discovery, it has brought together many researchers from various institutes to collectively and collaboratively improve our understanding of cancer. TCGA along with The Cancer Imaging Archive (TCIA) has stored the largest data in favor of cancer research, these data have also provided a crucial support for cancer radiogenomics studies, owing to their collection for several primary sites and a large amount of available data. The TCGA-TCIA so far has been able to characterize over 20,000 primary cancers along with 33 cancer types, which includes 10 rare cancer types.[3,4]
The Phase I of this project is a 3 years pilot study which aims to develop and test the research infrastructure based on the characterization of the selected tumors with poor prognosis which include brain, lung, and ovarian cancers. TCGA entered its Phase II in 2009 when its analyses had expanded to 30 different tumor types. For the successful running of the project, the NCI and NHGRI each invested $50 million for the Phase I, and the further funding for the Phase II was provided from different sources such as the American Recovery and Reinvestment Act.
THE CANCER GENOME ATLAS ORGANIZATION
Preferences
TCGA selection of tumors for research was preferred for those that met the criteria below:[2]
Prognosis was poor
Having impact on overall health
Passing patient consent standards
Sample availability (of high quality and quantity).
Inclusion of rare cancers with community and health professional support.
Steps
Figure 1 shows that TCGA was well-organized and involved several cooperating centers responsible for collection and sample processing followed by high-throughput sequencing and bioinformatics data analyses.

- Workflow and organization of The Cancer Genomic Atlas.
Data types
TCGA used high-throughput technologies based on microarrays and next-generation sequencing methods. The research structure contains many centers utilizing different platforms to provide global information of cancer genomics. The methods applied are illustrated in Figure 2.

- Analysis of genomic data: (1) It is created by National Cancer Institute (NCI) to collect and share with the public a large number of medical images of cancer form The Cancer Genomic Atlas (TCGA) cases. (2) It is a data storage of computed histology-based images of different tumor samples for TCGA cases. It is sponsored by Lawrence Berkeley National Laboratory. (3) It is an online interactive tool for viewing and annotating diagnostic and tissue slide images of different tumor types from TCGA project. (4) It is an analytical infrastructure created by the Broad Institute based on the needs of TCGA project. It provides a large amount of different quantitative algorithms such as GISTIC, MutSig, Clustering, and Correlation. (5) Used to identify and quantify the batch effects accompanying TCGA data set. (6) It is developed by NCI to integrate and display sample level genomic and transcriptional alterations in various cancers, from data from several cancer projects. (7) It is an open-access web-based tools developed and maintained by the UCSC Cancer Genomics Group to host, visualize, and analyze cancer genomics together with clinical data by utilizing genomic coordinate heatmaps. (8) Free to download, high-performance visualization tool introduced by Broad Institute for interactive exploration of large, heterogeneous, integrated data sets. (9) Developed by the Memorial Sloan-Kettering Cancer Centre for visualization, analysis, and download of large-scale cancer genomics data sets. So far, it stored data from 69 cancer genomics studies including DNA copy number data, mRNA and miRNA expression data, mutations, RPPA data, DNA methylation data, and limited clinical data related to survival.
MOLECULAR CHARACTERIZATION PLATFORMS
Since the discovery and introduction of TCGA, technology has rapidly advanced worldwide making it much easier for early diagnosis, treatment, and storing of data for future references. At the start of TCGA, microarray-based technologies were leading in the molecular characterization field. Shotgun sequencing of bacterial artificial chromosomes was the platform of choice for the human genome project, which became the starting step for the establishment of the reference human genome and a foundation for TCGA. For the next decade, due to TCGA demands for more scalable, low cost data, high-throughput sequencing rapidly developed and became accessible to researchers universally. At the end, TCGA was able to employ microarrays for profiling copy number variants, methylation, and protein expression and high-throughput sequencing for characterizing DNA and RNA,[2] Figure 3. Some of the technologies utilized and evaluated by TCGA over the years are listed below:
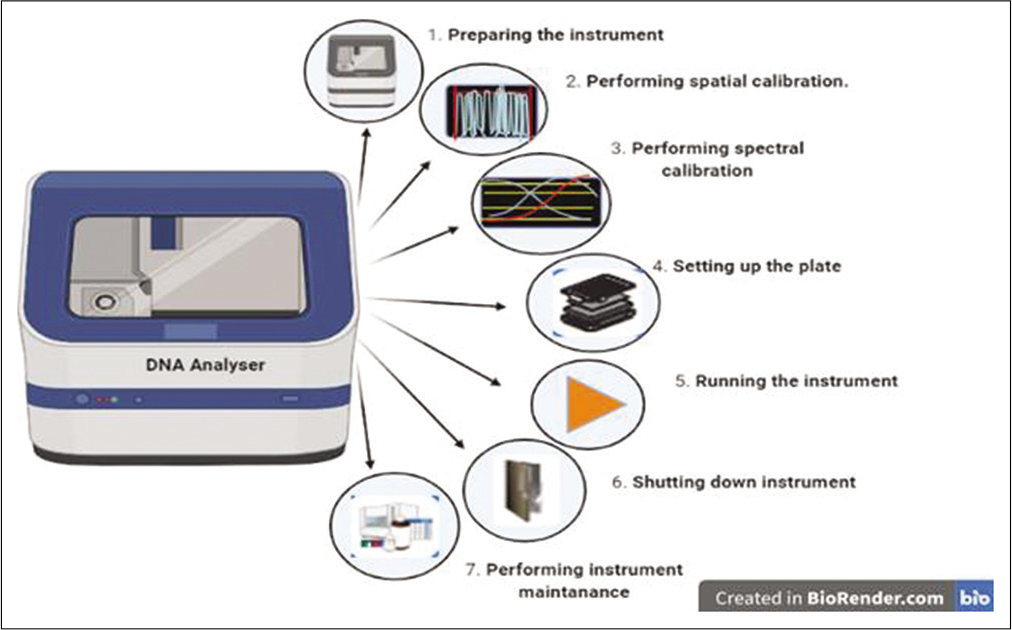
- DNA analyzer device and the steps involved in the DNA analyzer.
Broad Institute of MIT and Harvard – ABI (TCGA platform code); Applied Biosystems Sequence data (DCC Platform Name); DNA Analyzers (Instrument Support Materials); Primers (Sequence Download)
McDonnell Genome Institute at Washington University McDonnell Genome Institute at Washington University – ABI (TCGA platform code); Applied Biosystems Sequence data (DCC Platform Name); DNA Analyzers (Instrument Support Materials); Primers (Sequence Download)
Human Genome Sequencing Center at Baylor College of Medicine – ABI (TCGA platform code); Applied Biosystems Sequence data (DCC Platform Name); DNA Analyzers (Instrument Support Materials); Primers (Sequence Download)
U n i v e r s i t y o f N o r t h C a r o l i n a – AgilentG4502A_07_1(TCGA platform code); Agilent 244K Custom Gene Expression G4502A-07-1(DCC Platform Name); SurePrint G3 CGH+SNP Microarray (Instrument Support Materials); FASTA (Sequence Download)
University of North Carolina – AgilentG4502A_07_2 (TCGA platform code); Agilent 244K Custom Gene Expression G4502A-07-2 (DCC Platform Name); SurePrint G3 CGH+SNP Microarray (Instrument Support Materials); FASTA (Sequence Download)
University of North Carolina – AgilentG4502A_07_3 (TCGA platform code); Agilent 244K Custom Gene Expression G4502A-07-3 (DCC Platform Name); SurePrint G3 CGH+SNP Microarray (Instrument Support Materials); FASTA (Sequence Download)
Memorial Sloan Kettering Cancer Centre – CGH-1x1M_G4447A (TCGA platform code); Agilent SurePrint G3 Human CGH Microarray Kit 1x1M (DCC Platform Name); SurePrint G3 CGH+SNP Microarray (Instrument Support Materials); FASTA (Sequence Download)
Broad Institute of MIT and Harvard – Genome_Wide_ SNP_6 (TCGA platform code); Affymetrix Genome-Wide Human SNP Array 6.0 (DCC Platform Name); Genome-Wide Human SNP Array 6.0 (Instrument Support Materials); FASTA (Sequence Download)
McDonnell Genome Institute at – Genome_Wide_ SNP_6 (TCGA platform code); Affymetrix Genome-Wide Human SNP Array 6.0 (DCC Platform Name); Genome-Wide Human SNP Array 6.0 (Instrument Support Materials); FASTA (Sequence Download)
University of North Carolina – H-miRNA_8x15K (TCGA platform code); Agilent 8 x 15K Human miRNA-specific microarray(DCC Platform Name);Human mouse and rat miRNA Microarray (Instrument Support Materials); FASTA (Sequence Download)
University of North Carolina – H-miRNA_8x15Kv2(TCGA platform code); Agilent Human miRNA Microarray Rel12.0(DCC Platform Name); SurePrintG3 CGH+SNP Microarray (Instrument Support Materials); FASTA (Sequence Download)
Memorial Sloan Kettering Cancer Center – HG-CGH-244A (TCGA platform code); Agilent Human Genome CGH Microarray 244A (DCC Platform Name); SurePrintG3 CGH+SNP Microarray (Instrument Support Materials); FASTA (Sequence Download)
Harvard Medical School – HG-CGH-244A (TCGA platform code); Agilent Human Genome CGH Microarray 244A (DCC Platform Name); SurePrintG3 CGH+SNP Microarray (Instrument Support Materials); FASTA (Sequence Download)
Berkeley Lab – HuEx-1_0-st-v2 (TCGA platform code); Affymetrix Human Exon 1.0 ST Array (DCC Platform Name); Exon 1.0 ST Array (Instrument Support Materials); FASTA (Sequence Download).
CANCER DISCOVERIES FROM TCGA
By 2014, TCGA had analyzed more than 30 tumors. It’s multidimensional analyses and performances on various platforms provide better understanding of cancer biology, leading to improved cancer classification, development of new diagnostic methods, and therapeutic approaches. A few examples of discoveries through the TCGA are provided below:
Glioblastoma
It was the first cancer studied by TCGA. It is the most common primary brain tumor in adults. GBM is a fast-growing type of malignant brain tumor that is the most common adult brain tumor. GBM accounts for about 15% of all brain tumors and occurs in adults between the ages of 45 and 70 years. Patients with GBM have a poor prognosis and usually survive no more than 15 months following diagnosis.
The TCGA established and developed a novel subtype of GBM which affects younger adults and has an increased survival rate. These tumors are distinguished by a methylation signature which may account for the improved survival of these patients. The four distinct molecular subtypes of GBM respond differently to aggressive therapies: Proneural, neural, classical, and mesenchymal. The alterations of EFGR gene as well as a region on a chromosome containing MDM2 and CDK4 gene may be important to the development of GBM. The major gene mutations in the five genes provide new insights into the biology of this disease, these genes include NF1, ERBB2, TP53, PIK3RI, and TERT. The GBM mutations are enriched for chromatin modification genes.[3,4]
Ovarian serous adenocarcinoma
About 3% of all cancers is the ovarian serous adenocarcinoma which is specific to women where it occurs in the ovary of a woman’s reproductive system. Ovarian serous adenocarcinoma, the cancer studied by TCGA, is a type of epithelial ovarian cancer and it accounts for about 90% of all ovarian cancers. Due to improper diagnosis or late diagnosis, women are generally diagnosed at advanced stages. This type of cancer is due to mutations in the gene TP53 which is present in more than 96% of ovarian cases studied. A normal TP53 gene encodes a tumor suppressor protein that prevents cancer development, thus mutation in this gene leads to aggression of tumor development. Certain gene expression patterns correlate with poor or better survival. Patients with the poorest survival gene expression pattern lived 23% shorter period than other patients. According to TCGA, tumors associated with ovarian cancer categorized into four distinct subtypes according to gene expression and DNA methylation patterns. Patients with mutations in BRCA1 and BRCA2 genes have better odds of survival, and 21% of tumor cases had these mutations. Therapeutic opportunities for ovarian cancer lie in existing drugs targeting specific genomic errors.[5]
FUTURE PERSPECTIVES
TCGA is an assurance for thorough understanding of cancer. Till date, TCGA has produced an organized and a comprehensive catalog of cancer-specific genomic aberrations. The continuous advances in cancer genomics provided by the TCGA have introduced a new look through into the molecular biology of cancer. This application of high-throughput technology in combination with well-organized bioinformatic tools has contributed to determine the similarities and differences in the genomic architecture of each cancer and the various types of cancer, as depicted in Figure 4. The TCGA has provided a detailed genomic data allowing researchers worldwide an intense knowledge on cancer genetics, epigenetic profiles, and highlighting individual cancer biomarkers and drug targets. Furthermore, the conversion of cancer genomics into therapeutics and diagnostics provides a great stepping stone in the development and organization of cancer medicine. As the technology advances, there are many new changes occurring in TCGA to develop new bioinformatic tools that aim to prevent potential noise and to improve the resolution of the analysis. Recently, researchers are working on to develop an AI computer called Watson to help doctors in better diagnosis of patients.[6] These changes in research and medicine are sure to help the public in better treatment and early diagnosis of various cancer types.
Types of genome sequencing methods | Characteristics |
---|---|
1. RNA sequencing (RNAseq) | • Used for transcriptome profiling, and deriving strand information with very high precision • Rapidly identifies and quantify rare and common transcripts, isoforms, novel transcripts, gene fusions, and non-coding RNAs, among a wide range of samples, including low-quality samples • The TCGA deposits data containing information about both nucleotides sequence and gene expression |
2. MicroRNA sequencing (miRNAseq) | • It utilizes materials enriched in small RNAs • The detection of specific sets of short, noncoding RNAs that have the capacity to regulate hundreds of genes within or across diverse signaling devices is further carried out |
3. DNA sequencing (DNAseq) | • Nucleotides are determined within a DNA molecule, for providing information about DNA alterations such as insertions, deletions, polymorphism as well as copy number variation, mutation frequencies or viral infection events • The TCGA uses DNA sequencing systems based on sanger sequencing |
4. SNP-based platforms | • Analyses genome-wide structural variation across multiple cancer genomes • Array-based detection of single-nucleotide polymorphisms includes platforms able to define SNP, CNV, and loss of LOH across multiple samples |
5. Array-based DNA methylation sequencing | • TCGA utilizes DNA methylation assay based on the Illumina platform, assuring single base-pair resolution, high accuracy, easy workflows, and low input DNA requirements • This method is based on highly multiplexed genotyping of bi-sulfite-converted genomic DNA • The TCGA DNA methylation data files contain information for signal intensities, detection confidence, and calculated beta value for methylated and unmethylated probes |
6. Reverse-phase protein array | • Method for large-scale protein expression profiling, biomarker discovery, and cancer diagnostics • It is an antibody-based technique allowing for the analysis of more than 1000 samples with up to 500 different antibodies at a time • Th e TCGA DCC data include original images of protein arrays, calculated raw signals, relative concentration of proteins, and normalized protein signals |
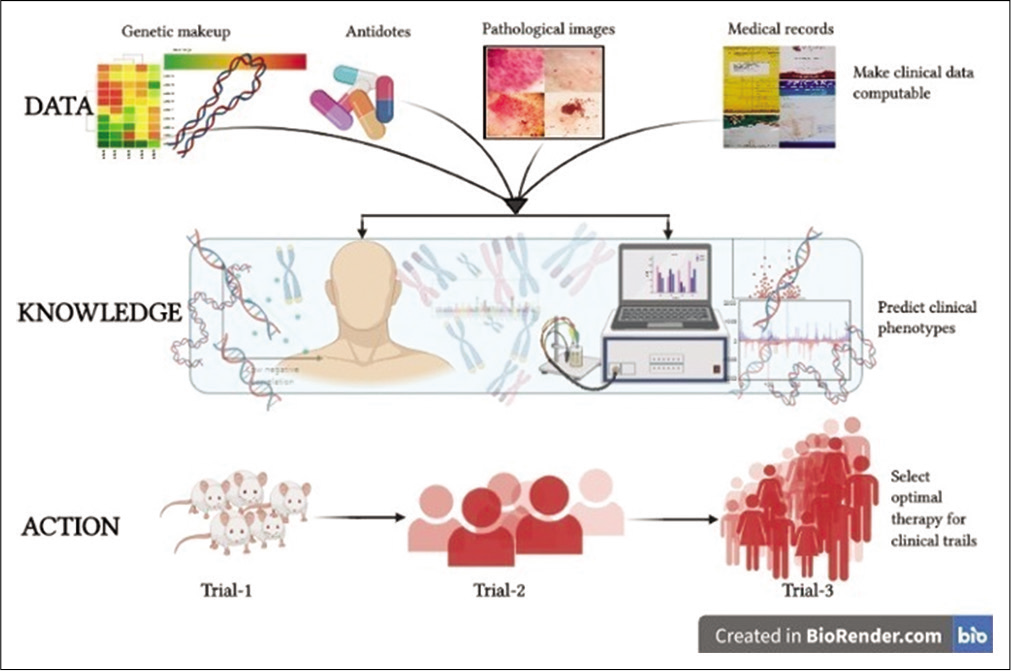
- Artificial intelligence is being implemented in various fields of science to assist medical professionals, to detect diseases at an early stage and diagnose with the best treatment possible. This also helps them to modify the traditional methods of treating a particular disorder in an appropriate way.
CONCLUSION
The merits and applications of the TCGA have been reviewed for the practicing clinical oncologist.
Acknowledgment
We would like to acknowledge Dr. Radhika Vaishnav for her mentorship and critical review of he manuscript. We also acknowledge our team members for valuable feedback.
Declaration of patient consent
Patient’s consent not required as there are no patients in this study.
Financial support and sponsorship
Nil.
Conflicts of interest
There are no conflicts of interest.
References
- Available from: https://www.cancer.org/cancer/cancer-basics/history-of-cancer/what-is-cancer.html [Last accessed on 2020 Sep 01]
- Available from: https://www.cancer.gov/about-nci/organization/ccg/research/structural-genomics/tcga/studied-cancers [Last accessed on 2020 Sep 01]
- Comprehensive genomic characterization defines human glioblastoma genes and core pathways. Nature. 2008;455:1061-8.
- [CrossRef] [PubMed] [Google Scholar]
- TCGA-TCIA impact on radiogenomics cancer research: A systematic review. Int J Mol Sci. 2019;20:6033.
- [CrossRef] [PubMed] [Google Scholar]
- Integrated genomic analyses of ovarian carcinoma. Nature. 2011;474:609-15.
- [CrossRef] [Google Scholar]
- Available from: http://www.ibm.com/smarterplanet/us/en/ibmwatson/index.html [Last accessed on 2020 Sep 01]