Translate this page into:
Driver mutations in oncogenesis
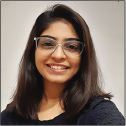
*Corresponding author: Shruti Morjaria, Department of Cell and Molecular Biology, Maharaja Sayajirao University, Vadodara, Gujarat, India. shrutimorjaria26@gmail.com
-
Received: ,
Accepted: ,
How to cite this article: Morjaria S. Driver mutations in oncogenesis. Int J Mol Immuno Oncol 2021;6(2):100-2.
All cancers occur due to changes in the DNA sequence of the genomes, and the genes whose mutation facilitates tumor growth are called driver genes. Cancer develops as a result of the accumulation of somatic mutation and other genetic alterations that impair cell division, checkpoints, etc., which results in abnormal cell proliferation and eventually tumorigenesis – such mutations are called “driver mutations” (the term driver mutation denotes mutation under positive selection within the population of cells), as illustrated in Figure 1. Along with driver mutations, there may be passenger mutation, which does not promote the growth of cancer cells or may not have any effect on the cells. Passenger mutations tag, along with the driver mutations because they are a consequence of simple cell division which will be carried along in the clonal expansion that follows, hence, will occur in all of the final cancer cells.[1,2]
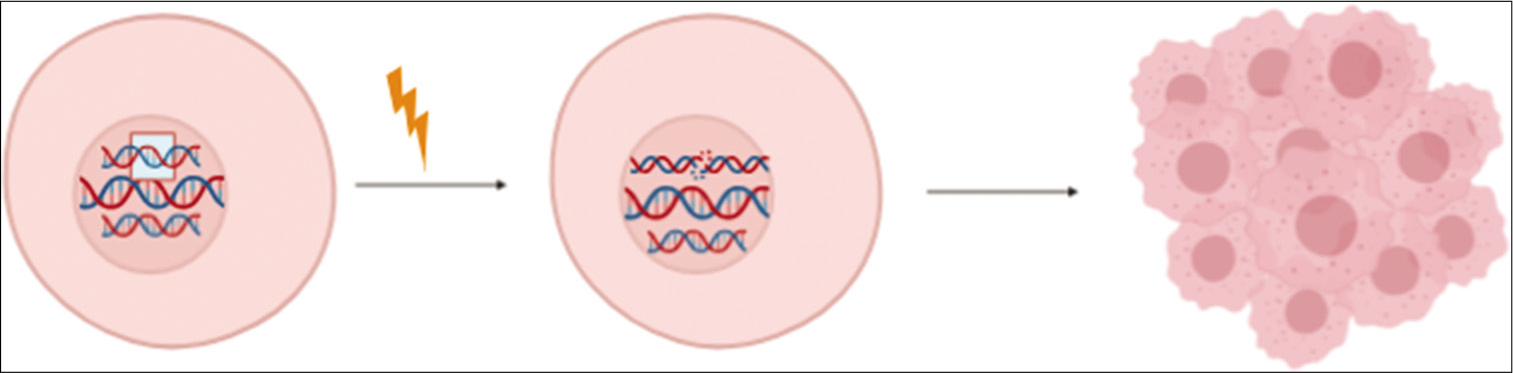
- Mutation in the driver genes of the genome causes the cell to become tumorigenic.
Cancer driver genes can be of two types (1) proto-oncogenes or (2) tumor suppressor genes, as shown in Figure 2. Genes such as BRAF, KRAS, and MYC are proto-oncogenes which require a gain of function mutation to become tumorigenic. Tumor suppressor genes such as TP53, PTEN, and CDKN2A are anti-proliferative and require inactivation of both alleles to become tumorigenic.[3,4]
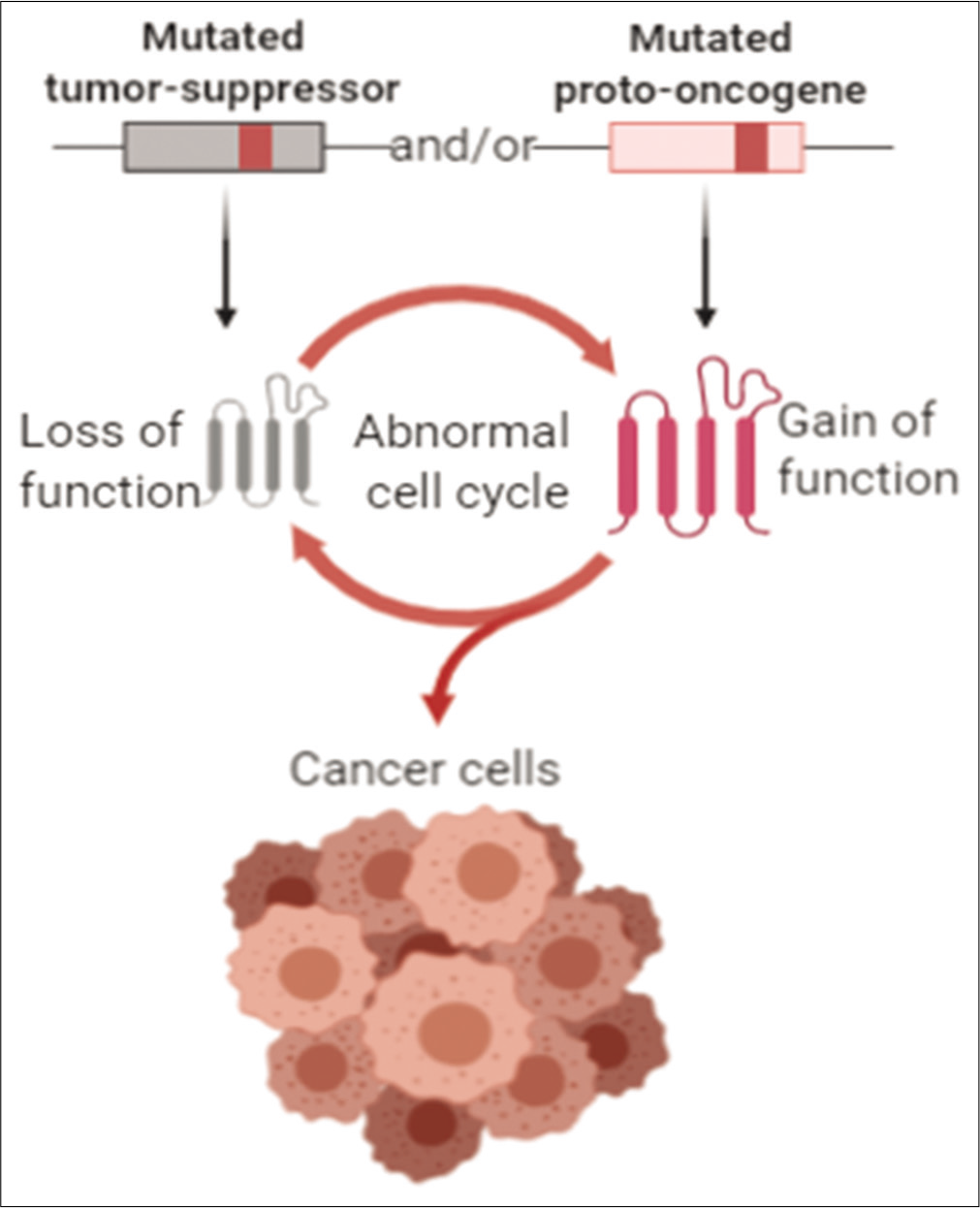
- Driver – gene classification.
Since there are many mutations occurring in the cancer cell genome, it is a challenge to identify specifically the driver mutation from all other passenger mutations. Large scale sequencing projects, such as (the Cancer Genome Atlas – a project to identify the complete set of DNA changes in many different types of cancers) and (Cancer Genome Project – aims to identify sequence variants/mutations critical in the development of human cancers), aim to identify all cancer-causing genes and accurate identification of all driver genes in the cancer of individual patients is of increasing interest for its application to precision in medicine. About 10% of driver mutation occur only in the germline; the other 10% of mutation occur in both somatic and germline, whereas 80% of the mutations are strictly somatic. The presence of driver mutation in germline indicates that a driver mutation can be acquired in earlier generations and become cancerous in the latter. This is possible because a cell may require multiple mutations to become cancerous which are acquired over time. As an instance, TP53 – tumor suppressor gene is a driver gene but acts as one only when a mutation has occurred in TP53 gene of both the alleles. Moreover, mutations may act as drivers only during certain stages of cancer.[5] Driver genes are not only part of the active genome (exons) but also a part of the introns.[3]
One of the methods for driver genes identification is based on the observation that drivers mutations target a region in a protein (e.g., region of phosphorylation) and the mutations will be clustered near to each other in a linear sequence of the gene. As a result, genes that are clustered rather than distributed evenly can be classified as driver mutations. An excess of mutations is a signature of positive selection, and genes that are mutated more frequently than expected are possible driver genes. Driver mutations are more prominent across multiple independent tumors. This concept has given rise to a class of methods that seek to identify the region of DNA that has excess of mutation relative to an expected base rate due to mutational processes.[3]
At present, about 568 genes from 28,000 tumors and 66 types of cancers have been identified as driver genes, which, on being mutated, have the ability to cause cancer. It has been observed that driver genes are highly specific with their mutations and can trigger only a few tumor types out of which, 2% of the driver mutations are responsible for causing more than 20 types of cancer. It is very significant to know, identify driver genes and its tumorigenic capacity to know the potential targets for therapy, that is, antibodies or other inhibitors to treat cancer patients.[6]
After having known so much about driver genes, there are still challenges that must be looked upon. Majority of driver genes are identified by their frequency of occurrence; hence, drivers with higher frequency are identified, but those with rare occurrence are not. Rare driver gene mutations are likely to be present in less than 1% of cancers. Scientists have considered a theoretical basis to explain why some driver mutations are rare. They explain that, a protein may be present in an inactive state and high-frequency or low-frequency tissue specific driver mutations shift the protein state from inactive to active. It is argued that occurrence of few driver mutation is rare because without an additional mutation, the protein activation is not sufficed. There is a huge possibility that rare drivers are clinically diagnosed but not identified using computational software, which is an emerging challenge.[7] It is now known that non-coding genes also contribute to the list of driver mutation, but about ∼99% of our genome is non-coding. Although we have the locations of these elements identified, they are fairly large sequences. However, this is partly because our techniques for determining the positions of these elements are imprecise – their real functional territory is often smaller than that annotated and hence to identify driver mutations in the non-coding regions, which is a challenging task.[8]
Recently, a group of Yale researchers have developed a mathematical model which specifically identifies driver mutations and further estimates their tumor growth pattern. The model is based on the frequency of the mutations. A mutation with higher frequency must have occurred early in the pool of mutations, while others may be a part of the collateral damage in the tumor. The mathematical model uses the frequency of the mutation that has occurred exactly before the driver mutation to identify the driver and where the tumor first emerged from. Conventionally, to identify driver mutations, cohort studies, which include large number of samples to be examined, are required. The involvement of cohort studies makes the assessment of how a tumor develops more complicated to attain. The model developed does not require cohorts, but only one tumor to be very deeply sequenced. The approach incorporated requires sequencing of the same location in the genome several times to identify rare variations. To check the effectiveness of the model, the same was applied to 993 tumors obtained from Pan-Cancer Analysis of Whole Genomes Consortium to predict the presence, time of occurrence, and effect of driver mutation. Instead of looking at a large database, we can look at a single tumor and deep sequence it; this is the novelty of the model. The model’s effectiveness provides reasons to be optimistic about its future application and make a difference in the future of cancer treatments.[9]
Declaration of patient consent
The authors certify that they have obtained all appropriate patient consent.
Financial support and sponsorship
Nil.
Conflicts of interest
There are no conflicts of interest.
References
- Mutations: driver versus passenger In: Boffetta P, Hainaut P, eds. Encyclopedia of Cancer (3rd ed). Oxford: Academic Press; 2019. p. :551-62.
- [Google Scholar]
- Driver and passenger mutations in cancer. Annu Rev Pathol. 2015;10:25-50.
- [CrossRef] [PubMed] [Google Scholar]
- A compendium of mutational cancer driver genes. Nat Rev Cancer. 2020;20:555-72.
- [CrossRef] [PubMed] [Google Scholar]
- Why are some driver mutations rare? Trends Pharmacol Sci. 2019;40:919-29.
- [CrossRef] [PubMed] [Google Scholar]
- Cancer genomics: Less is more in the hunt for driver mutations. Nature. 2017;547:40-1.
- [CrossRef] [PubMed] [Google Scholar]
- Estimating growth patterns and driver effects in tumor evolution from individual samples. Nat Commun. 2020;11:732.
- [CrossRef] [PubMed] [Google Scholar]